Detecting Active Tuberculosis Bacilli - 2024
Daily submission limit: 1
- Contest starts on Friday, March 1, 2024. We recommend users to sign up on the platform earlier if possible. You will be able to do the tutorials and look at the data sets any time.
- Contest ends on Monday, April 1, 2024.
Table of Contents
- Overview
- Task
- Baseline Score
- Prizes and Compute Credits
- Dataset
- Important Dates
- Rules
- Scoring
- Frequently Asked Questions
- Host organizations and Acknowledgments
Overview
Tuberculosis is one of the leading infectious causes of death worldwide 1. Each year, millions of individuals contract and develop active TB without knowing 2. Case identification and treatment are the primary methods for controlling spread as there is no effective TB vaccine for adults. Unfortunately, delays in diagnosis are common, especially in resource-limited settings, and can worsen individual outcomes and perpetuate transmission of the disease 3,4. Without a timely diagnosis, patients needing treatment would head home from a clinic without knowing they were positive. If they miss their follow up, they would not learn about their diagnosis and nor would they start their treatment. Automated TB diagnosis could play a role reducing the loss of follow up and get patients to be treated more timely. Automated digital microscopy has been proposed as a cost-effective solution 6,7. An automated algorithm that could reliably detect mycobacterium on samples from patients with suspected TB would lead to more accurate and timely diagnosis of active TB in many places that currently have barriers to both.
Task
This challenge was developed to catalyze the development of algorithms that aid in the diagnosis of active Tuberculosis through the detection of TB bacilli on patient samples. The dataset contains thousands of microscopic images taken from samples collected across Asia. The samples are smears of respiratory sputum that have been stained in a way that make the TB bacilli appear in a pinkish hue (acid fast bacilli).
The images in this dataset have been reviewed and labeled with whether they contain TB bacilli. The labeling process involved two or more certified medical technicians reviewing each image and giving them a positive or negative label for presence of TB bacilli. The images with discrepancies in the labeling were removed from the dataset. Furthermore, all the positive images came from patients with culture proven tuberculosis.
The dataset has been divided into a training dataset and a holdout dataset. The contestants will have access to labels of the training dataset and expected to make predictions for the images in the holdout dataset.
The goal of this challenge is to use the provided labels to predict whether there are any TB bacilli present in the microscopy images of the holdout dataset.
This contest will take a straightforward approach of grading algorithmic predictions against the human generated labels of holdout dataset as if it were the ground truth. But of course, because the images are labeled by humans, there may be errors: humans can have false positives (flag a bacillus when none is present) and false negatives (miss a bacillus somewhere in the image). Using algorithmic predictions to identify human errors and re-label images could be an interesting avenue of research and potential use of this dataset but is beyond the scope of this data challenge.
The leaderboard placement will be determined based on the area under the precision recall curve (PR-AUC) for predictive accuracy of the presence of TB bacilli. This metric was chosen because the dataset is imbalance, with only about 5% positive observations.
Please refer to the full version of the dataset documentation.
Baseline Score
The Nightingale team created to baselinea baseline model to set a minimum minimum average precision for the performance metric of the data challenge. Submissions that beat this baseline will be eligible for prizes subject to the rules below.
The approach was to take advantage of the acid-fast staining of the smears and the rod-shape of the TB bacilli. The first step was to identify regions of interest (ROI) on the images using the color and morphology. These regions were then divided into 224 x 224 patches that would be used to train a convolutional neural network (CNN).
The trained model was then evaluated on the holdout dataset. The ROC-AUC was 0.88 and the PR-AUC was 0.51. The average precision score is used to calculate PR-AUC. The graphs of these curves are included below.
Figure 1. The performance of the baseline model.
Prizes and Compute Credits
Compute Credits
Each team will be allocated compute credits worth $250 when they join the contest and request them. Active teams may request additional tranches of $250 of free compute credits when they have less than $50 balance, up to a maximum of $1,000 per team for the duration of the contest. Complimentary compute credits will expire at the end of the contest. Teams may also pay for their own compute credits beyond this limit – they will not expire at the end of the contest.
Prizes
Winners will be decided based on substantially original work with high PR-AUC on the leaderboard scores that beat the baseline model’s PR-AUC. The leaderboard placement will be determined based on the area under the precision recall curve (PR-AUC).
All prize winning entries are subject to rules governing the prizes and the contest. The top two winners will receive cash prizes of $3,000 and $2,000 respectively.
Please see the rules for US export controls and tax reporting/withholding information.
Dataset
The dataset contains images of tuberculosis smears used to detect the presence of acid-fast bacilli in sputum samples. This dataset is a subset of tuberculosis smear images that Wellgen Medical collected from across Asia to train and validate their smear microscopy automated system 8,9. The slides mostly come from Taiwan, and partially from China, India, and Japan. The slides are digitized using a microscopic scanner and are subdivided into images with the resolution of 2448x2048.
Figure 2. An example of an image. The dataset images do not have bounding boxes.
All positive smear images in the dataset come from sputum slides with culture-proven tuberculosis. Many images are generated from a single slide and not all images contain TB bacilli. However only the images with TB bacilli are included in the dataset with the positive label. These bacilli are identified with Wellgen Medical’s AI algorithm and then double checked by two licensed medical technicians.
In the dataset all negative smear images come from sputum from patients with negative culture results.
~/datasets/tb-wellgen-smear/supplementary/contest/
Labels: The label for this dataset is the the presence of TB bacilli. 5.3% of the images in training dataset are positive. The positivity of this label is not dependent on the quantity of TB bacilli. The presence of a single bacteria will receive a positive label.
Dataset splits: The dataset has been split randomly. It was a 75/25 split with the 25% holdout used for validation purposes. The 75% data and its labels have been made available on the platform
Table 1. Training Dataset
Count | Percentage | |
---|---|---|
Total Images in Training Dataset | 75,087 | |
Images given a positive label (TB-Positive) | 3,976 | 5.3% |
Images given a negative label (TB-Negative) | 71,111 | 94.7% |
Important Challenge Dates
ATTENTION: The contest ends on April 1, 2024.
- Friday, March 1, 2024 - start date
- Monday, April 1, 2024, 11:59PM EDT (New York time) - contest deadline
- April 2024 - results announced.
Rules
-
Registration required. Only registered Nightingale OS users may participate in the contest.
- Registration is open to anyone worldwide who has an active affiliation with an accredited academic institution.
- Your registration must be approved by Nightingale before you can access contest data or any other Nightingale OS resources.
- You can only sign up for one account.
- You must abide by all Nightingale Terms of Service. In particular, no portion of a Nightingale dataset may be downloaded.
-
Teams and collaboration
- Size limit. Teams can be any size. As a general rule, contests often limit team sizes to about 5. Because this is a multi-disciplinary research area, Nightingale recognizes that teams may be larger from time to time.
-
No merges. You can only be a member of one team during this challenge.
- If you entered the competition as an individual, you may join someone else’s project only if you have not submitted any entries for scoring during the contest’s current phase.
- No sharing. Sharing code between teams is prohibited unless the information shared is free and publicly available.
- Public discussion. You may not publicly describe the methodology you are using for the competition until after the submission deadline for a given phase. You may describe your methodology in the context of other datasets as long as you don’t also indicate this is the methodology you used in competition.
- Publication. Please use the recommended citation found on the dataset documentation page. Although the dataset is available to all Nightingale users, please do not submit contest-related work to other conferences or journals until the current contest has ended.
-
Scoring
- Predictions CSV. Teams submit entries for scoring in the form of a predictions file. Nightingale will score the entry according to the methodology described in the Scoring methodology section.
- Entry limit. Each team can submit 1 entry per day.
- Public leaderboard. During the competition period, each team’s current ranking will be visible on the competition public leaderboard. After the end date, the leaderboard will reveal each team’s score and model description.
- High score. The best score from all your team’s submissions will be used in the leaderboard, along with your description of that entry, if any. See Scoring for details.
- Tiebreaker. In the event of a tie, the first submission will outrank subsequent submissions.
- Submission requirements. Nightingale may make efforts to help you validate your predictions file and fix any issues before scoring. However, invalid submissions may result in no score and may count against your team’s entry limit.
-
Resources
- Use of non-public data or software. Use of free and publicly available external data is allowed, including pre-trained models. If you use any proprietary software or data that is not free and publicly available, such as a pre-trained model using private data, then you should declare this by including “[NON-PUBLIC]” in your Nightingale project description field. Rankings and scores for teams using non-public resources will be published on the leaderboard and encouraged to contribute to the conferences but will not be eligible for any prizes.
- Billing. Nightingale OS provides both free and non-free compute resources to contest participants. To support participants with the cost of non-free compute servers, Nightingale allocates a limited amount of free compute credits to the teams on sign up. Additional free compute credits will be allocated periodically based on availability of credits and each team’s activity. Teams are responsible for all additional costs incurred for non-free resources after they consume the free compute credits.
-
Prizes
- Review of entries and Deadlines. All top scoring entries are subject to Nightingale’s review for compliance with guidelines and quality of results. After the deadline, the organizers will add the reviewers to the leading projects so that their submissions can be reviewed for conformance with the rules for awarding prizes. Because the goal of this contest is to promote research and to stimulate interest in these kinds of applications, we could extend the deadline in order to get broader participation and high quality submissions. We will let everyone know in advance if we do change any key dates.
- Publishing and Licensing of work. To be eligible for prizes, the teams must publish their winning solution on their public Github code repository as open source under the Apache License v2 or an Apache-compatible open source license. The license must be added to your project files by the deadline to be eligible - see here for an example of how to do this. Third-party resources, if used, must be freely available to the public, and must not form a significant part of the solution as determined by the judges. This clause is intended to promote the repeatability and reproducibility of science. If a team is unable to comply with this clause, they may still compete and will be placed on the leaderboard, but they will not be eligible for prizes.
- Export control. Prize money is subject to US export control regulations. Teams in countries prohibited by US export control regulations will not be eligible for prizes.
- Tax reporting and withholding. ALL TAXES IMPOSED ON PRIZES ARE THE SOLE RESPONSIBILITY OF THE WINNERS. Payments to potential winners are subject to the express requirement that they submit all documentation requested by competition organizers for compliance with tax reporting and withholding regulations. Prizes will be net of any taxes that the competition organizer is required by law to withhold. Foreign residents (as defined by US tax regulations) may be subject to mandatory 30% tax withholding. If a potential winner fails to provide any required documentation or comply with applicable laws, the Prize may be forfeited and the organizer may select an alternative potential winner. Any winners who are U.S. residents may receive an IRS Form 1099 in the amount of their prize. Any winners who are foreign residents may receive an IRS Form 1042-S in the amount of their prize and the tax amount withheld.
Scoring
Scoring Methodology
The area under the precision recall curve (PR-AUC) has been chosen to measure the performance of the contestants’ algorithms. This metric has been chosen, because the dataset is imbalanced with only about 5% of the data having a positive label.
Nightingale has randomly sampled the images to create a holdout subset of the data. You can see the images in the dataset directory in the holdout subdirectory, but of course, the labels are excluded.
~/datasets/tb-wellgen-smear/supplementary/contest/images-holdout
To submit an entry, predict the presence of TB bacilli for each image and write results to a CSV file.
Predictions CSV file format
-
Filename and location
-
The file needs to be located in your project directory. Specifically, a predictions file should be in
~/project
or any subdirectory. - The predictions file can have any name.
- For subsequent submissions, it doesn’t matter whether you use the same file or create a new one.
-
The file needs to be located in your project directory. Specifically, a predictions file should be in
-
CSV format
- The CSV file should have no header row.
- The Image_id is the file name of the image without the file extension.
- The Probability must be a float between 0 and 1, inclusive.
- Each line should have the following schema:
Column index | Contents | Data type |
---|---|---|
0 | Image_ID | string |
1 | Probability | float in range [0,1] |
Example CSV
47ba1eb2,0.67326
e4235769,0.27895
d9bd5e69,0.0064058
...
note: no header is included
Submission description (recommended)
- You may optionally add a description for each submission. You don’t get to change the description later. We strongly recommend you use this field to note the version of the model that generated it, so that scores can be traced back to a particular model.
- You will see descriptions in your Submissions list inside your team’s Nightingale project.
- The public will see the description for your best submission after the contest end date.
Frequently Asked Questions
I’m new to Nightingale. How can I learn to use the platform quickly?
Please see the quick start tutorial here. The tutorial notebook is also available inside the platform - you can copy, run, and modify the notebook hands-on to learn to use the platform quickly.
How to request complimentary compute credits?
To request these compute credits, teams should submit a help request from the platform or email support@ngsci.org, mentioning their project id. Complimentary credits will expire at the of the contest. Please see the details on the amount of free compute credits here.
How to get additional paid compute credits?
If a team wants to pay for additional compute credits, they may generate an invoice for a specific dollar amount and have it mailed to the payee’s email address. This invoice email will contain an electronic link for online payment using a credit card. On successful payment, these credits will be added to the project. These paid compute credits will not expire at the end of the contest, and we are able to transfer unspent compute credits to a new project created by the same user.
How to submit an entry?
Submit your predictions file inside any instance in your Nightingale project:
>>> import ngsci
>>> ngsci.submit_contest_entry("path/to/your.csv", description="our model")
(<Result.SUCCESS: 1>, 'success')
After you submit a file, Nightingale will attempt to validate the schema of your CSV. If it fails validation, you will see one or more errors in the Submissions tab in your Nightingale OS project (not in your JupyterLab editor). Any validation errors detected automatically will not count against your submission limit.
After a predictions file passes validation, it will be submitted for scoring. The result will be posted in your project Submissions view, and if the result is a new high score for your team, the public leaderboard will also be updated.
Can I see a sample submission?
A sample CSV file and notebook are located in ~/datasets/tb-wellgen-smear/supplementary/contest/sample-submission/
. The sample CSV is in the proper format and contains all the image_id
‘s needed for a submission. The notebook describes the process of submitting an entry.
How should I submit my model?
Teams that are in contention for winning prizes will be expected to share their projects with Nightingale staff within 3 days after the submission deadline. More precise instructions will be given to the top teams a week before the deadline.
Expected to include:
- The notebooks or scripts used to train the model
- The weights or serialized model used for winning submission, which can be used to reproduce submission results
- A Open-source license
Here are some tutorials for saving weights and models in PyTorch and Tensorflow.
How to enter the contest and form a team?
After you have registered for Nightingale and a Nightingale admin has admitted you, then you can enter the currently active contest. For example: Detecting Active Tuberculosis Bacilli - 2024
.
When you enter the contest, a new, empty project is created for you and associated with this contest. This project is your team workspace.
- Your project (team) name and description will be publicly visible on the leaderboard.
- Add teammates by making them member of your project.
- Only registered users are eligible to be added to your project/team.
- You can only be a member of one team per contest.
Host organizations
This is a challenge jointly hosted by Nightingale Open Science and Wellgen Medical.
Nightingale Open Science is a platform connecting researchers with de-identified, cutting edge medical datasets. The Nightingale OS team works closely with health systems around the world to create and curate datasets of medical images linked to ground-truth labels, and make them freely available to academic researchers. Nightingale OS launched at the 2021 NeurIPS conference with five anchor datasets spanning different disease areas.
Established in 2016, Wellgen Medical has developed automated microscopy technology used to detect disease. In order to train their own AI algorithms, Wellgen Medical has collected large amounts of disease samples. Partnering with Nightingale Open Science, Wellgen Medical is sharing their TB smear collection with the broader scientific community in order to fight tuberculosis.
Together, our teams are thrilled to collaborate on this contest to spur collaboration and competition in the field of computational medicine.
Acknowledgements
We thank our generous funders: Schmidt Futures, The Gordon and Betty Moore Foundation, and Ken Griffin. We would also like to acknowledge the team that created and conceived of this dataset, and worked with us to make this challenge possible: Yusen E. Lin, PhD, MBA, Founder of Wellgen Medical.
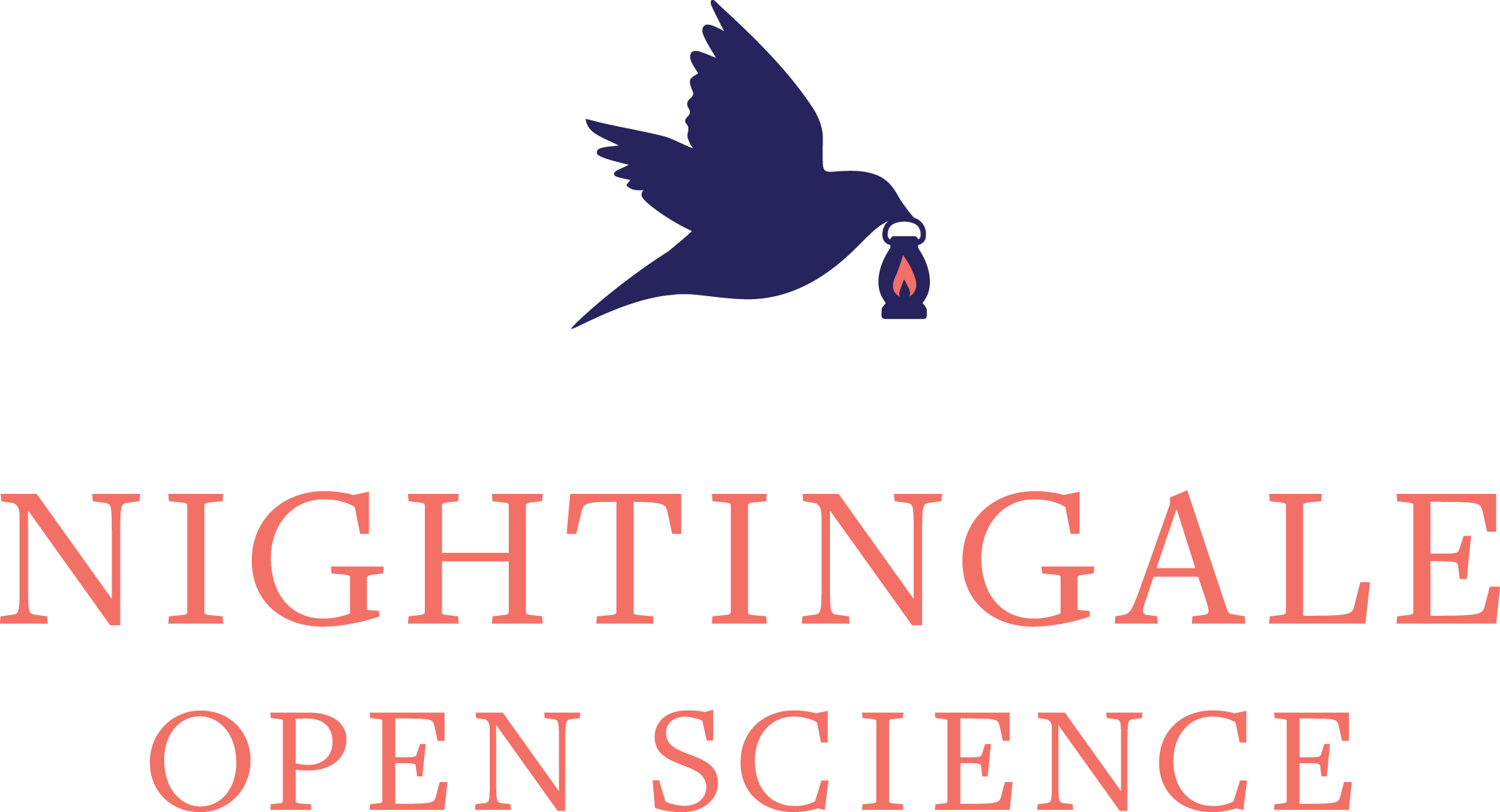