AIM-AHEAD Health Equity Data Challenge 2023
Daily submission limit: 1
- Participants must meet AIM-AHEAD eligibility criteria and sign up on AIM-AHEAD Connect before joining this challenge. Please see Rules (items 1.1 and 5.3) for more information.
- Contest starts on Monday May 22, 2023. We recommend users to sign up on the platform earlier if possible. You will be able to do the tutorials and look at the data sets any time.
- Contest ends on Friday June 23, 2023.
- Challenge winners will be invited to present at the NIH AIM-AHEAD conference in Bethesda, Maryland from August 1-3, 2023.
- Contestants will be granted compute credits and winners will be awarded cash prizes by AIM-AHEAD.
Table of Contents
- Overview
- Goals and Applications
- Task
- Past Challenges
- Baseline Score
- Prizes and Compute Credits
- Dataset
- Important Dates
- Rules
- Scoring
- Frequently Asked Questions
- Host organizations and Acknowledgments
Overview
Every year, 40 million women get a mammogram; some go on to have an invasive biopsy to better examine a concerning area. Underneath these routine tests lies a deep—and disturbing—mystery. Since the 1990s, we have found far more ‘cancers’, which has in turn prompted vastly more surgical procedures and chemotherapy. But death rates from metastatic breast cancer have hardly changed.
When a pathologist looks at a biopsy slide, she is looking for known signs of cancer: tubules, cells with atypical looking nuclei, evidence of rapid cell division. These features, first identified in 1928, still underlie critical decisions today: which women must receive urgent treatment with surgery and chemotherapy? And which can be prescribed “watchful waiting”, sparing them invasive procedures for cancers that would not harm them?
There is already evidence that algorithms can predict which cancers will metastasize and harm patients on the basis of the biopsy image. Fascinatingly, these algorithms also hone in on features that humans neglect, for example, the nature of the non-cancerous tissue surrounding the tumor. But to date, the datasets linking biopsy images to patient outcomes—metastasis, death—have been far smaller than what is needed to apply modern approaches.
Many studies did not have sufficient participation of minority patients due to health disparities and inequities. It has been well established that inclusion of minority race and ethnicity patients is important for the study results to be applicable to a diverse population. This need has been crucially recognized for treating patients with breast cancers.
Breast cancer health disparities and inequities exist on many levels, including race, ethnicity, socioeconomic status, geography, and access to healthcare. For example, Black women are more likely to die from breast cancer than white women, despite having a lower incidence of the disease. This is due to a combination of factors, including differences in access to healthcare, late diagnosis, and more aggressive forms of the disease.Triple negative breast cancers (TNBC) are characterized by the absence or low levels of estrogen receptors (ER), progesterone receptors (PR), and human epidermal growth factor 2 receptors (HER2) on the tumor cells. According to the American Cancer Society, tThey constitute about 15% to 20% of breast cancers and are among the hardest subtype to treat. They have higher incidence in African American and Hispanic women, with African Americans facing worse outcomes than other race and ethnic groups.
Goals and Applications
Cancer staging is a complex, multidisciplinary task: while it does take into account some features of the biopsy, it also integrates a wide variety of external information: the size of the lesion biopsied, its appearance and location on imaging, and a variety of other tests (imaging and more) to determine whether the cancer has spread to other locations in the body. This important contextual information, most of which is not present in the whole slide image, serves as our ground-truth label for the challenge. By linking features of the whole slide image to this label, algorithmic approaches have the potential to find new sources of signal—beyond the tubules, atypical nuclei, and cell division markers pathologists consider today—that can identify patients with benign or deadly cancers.
The goals of this NIH AIM-AHEAD-Nightingale challenge are four fold
(a) Community engagement- to offer a platform in the form of a data challenge, in order to bring together a diverse group of individuals, with the goal of developing solutions that address health equity using AI.
(c) Collaboration - To empower the participants of this challenge to examine the effect of healthcare disparities, by using whole slide images and race information to predict the severity of the breast cancer; and assess the potential impacts on marginalized communities
(c) Education - To raise awareness of the challenges related to health inequities
(d) Application - Can these innovative the proposed AI solutions be implemented in the real world to promote positive change?
Building on successful work in this challenge, a particularly interesting next step is to identify predictable “outliers”: patients whose cancer is far more—or less—benign that it appears to the pathologists. Researchers at Providence, who have access to rich and granular data on pathologists’ judgments, are eager to collaborate on this exciting follow-on work.
Task
NIH AIM-AHEAD and Nightingale developed this challenge in order to catalyze the development of algorithms that find new signals in digital pathology images, ultimately providing new insights into which minority patients may be at risk and need preventive treatment.
The goal of this challenge is to predict the stage of minority patient’s cancers, using only the slide images generated by a breast biopsy.
The leaderboard placement will be determined based on the area under the ROC curve for predictive accuracy in minority (defined as race other than Caucasian or ethnicity is Hispanic) patients. Additional prizes may be awarded for outstanding submissions with high accuracy of predicting cancer stage in patients with triple-negative breast cancers, irrespective of race or ethnicity.
Please refer to the full version of the dataset documentation as you get started to learn more about the cohort and key variables for this challenge including mortality and cancer stage.
Past Challenges
Nightingale along with partners Providence St. Joseph Health (Providence) and the Association for Health Learning and Inference (AHLI) have hosted two breast cancer prediction challenges. The source code of the winning solutions have been published to support reproducible as well as collaborative research. The results and source code of winning solutions are published here for reference. The participants may use these for educational purposes, but their submissions must substantially be their own work.
Baseline Score
Nightingale team used CLAM‘s out of the box model to calculate the baseline score using only the biopsy images. CLAM identified the regions of slides that contain tissues and uses transfer learning from a pre-trained model. CLAM is a method that uses weakly supervised learning using slide-level labels to classify whole slide images (WSI). Using CLAM’s out of the box model, our baseline model obtained an AUC of 0.707
The model used to calculate the baseline score is available on the Nightingale platform in the directory. Participants may use this baseline model as a tutorial to understand how to access the data set, and to train and validate the model. However, their submissions must substantially their own work to be eligible for prizes.
Prizes and Compute Credits
AIM-AHEAD has announced prizes up to $5,000 so far, and free compute credits worth up to $20,000 for this challenge.
Compute Credits
Each team will be allocated compute credits worth $250 when they join the contest and request them. Active teams may request additional tranches of $250 of free compute credits when they have less than $50 balance, up to a maximum of $1,000 per team for the duration of the contest. Complimentary compute credits will expire at the end of the contest. Teams may also pay for their own compute credits beyond this limit – they will not expire at the end of the contest.
Prizes
Winners will be decided based on substantially original work with high AUC on the leaderboard scores that beat the baseline model’s AUC. The winning teams will be awarded total cash prizes and will be invited to attend the AIM-AHEAD conference in Bethesda, MD. The winning teams will be awarded cash prizes as follows:
First place: $3,000 Second place: $1,250 Most innovative solution in R: $750
All prize winning entries are subject to rules governing the prizes and the contest.
The leaderboard placement will be determined based on the area under the ROC curve for predictive accuracy in minority (defined as race other than Caucasian or ethnicity is Hispanic) patients. Information on travel support for winners will be announced soon.
Dataset
This dataset contains whole slide images from 1,000 breast biopsies, in 842 patients, over the years 2014 to 2020. For our purposes, an observation in this dataset corresponds to a biopsy (i.e., performance in the hold-out set will be evaluated at the biopsy level). The contest content can be found in the contest directory.
~/datasets/brca-psj-path/contest-phase-2
Images: Each biopsy generates between one to one hundred physical slides (processed with hematoxylin and eosin stain). The slides have been digitized at 40x magnification with a Hamamatsu slide scanner, yielding a whole slide image. These images have a resolution around 100,000 x 150,000 pixels, and are stored as a single NDPI file (average size ~2GB). A NDPI file is a TIFF-like file, and libraries like openslide can be used to interact with them. The 1,000 biopsies of this dataset generate 10,856 whole slide images, with a median of 5 WSI per biopsy.
Labels: The primary label is the cancer stage associated with a biopsy. 94% of staging judgments in this dataset are made within one month of biopsy).
Dataset splits: The dataset has been split randomly at the patient level. It was a 75/25 split with the 25% holdout used for validation purposes. The 75% data was then subsampled in order to increase minority patients representation, and this subset of 1,000 biopsies have been made available for phase two of this competition. Refer to Table 1 for what is expected to be made available.
Table 1
Train | Holdout | |
---|---|---|
N biopsies | 1000 | 1077 |
N patients | 842 | 856 |
N images | 10856 | 16899 |
N biopsies with stage | 1000 | 886 |
0 | 18% | redacted |
I | 39% | |
II | 20% | |
III | 17% | |
IV | 6.0% | |
N unstaged biopsies | 0 | 191 |
Model performance measurement: The primary metric we will evaluate model performance is prediction of cancer stage, among staged cases in the holdout set. More detailed information on the exact scoring methodology is below. The holdout set contains staged and unstaged biopsies (see Table 1), but only staged biopsies will be used to calculate the primary Challenge metric. We will award additional prizes for other aspects of performance, reflecting both clinical utility of models and Nightingale Open Science’s commitment to equity.
You are free to use images from unstaged cases in any way you’d like in the training process. You are also free to use any other available information in the training process: detailed demographic information including age, sex, and self-reported race; and other information about the cancer and its progression beyond stage, including mortality and ICD codes for metastatic disease (though keep in mind the many caveats of this information, as noted for example here). However, note that none of this contextual information will be provided in the holdout set—only the slide images. Please refer to the full version of the dataset documentation as you get started to learn more about the cohort and key variables.
Important Challenge Dates
ATTENTION: The contest ends on June 23, 2023.
-
Monday, May 22, 2023 - start date
-
Friday, June 23, 2023, 11:59PM EDT (New York time) - contest deadline
-
July 2023 - results announced.
-
August 1-3, 2023 - AIM-AHEAD conference in Bethesda, MD.
Rules
-
Registration required. Only users who meet AIM-AHEAD eligibility criteria are eligible to participate.
- Users meeting AIM-AHEAD criteria must first register for the challenge through AIM-AHEAD Connect.
- You must also register with Nightingale and have your registration approved before you can join the contest.
- After successfully completing the above two steps, you may join the contest from this page.
- You must abide by all Nightingale Terms of Service. In particular, no portion of a Nightingale dataset may be downloaded.
- You may invite other AIM-AHEAD and Nightingale registrants who meet the above criteria using their email address to join your team. We strongly suggest that each team include some members from communities underrepresented in data science and artificial intelligence and machine learning. For examples, see here.
-
Teams and collaboration
- Size limit. Teams can be any size. As a general rule, contests often limit team sizes to about 5. Because this is a multi-disciplinary research area, Nightingale recognizes that teams may be larger from time to time.
-
No merges. You can only be a member of one team during this challenge.
- If you entered the competition as an individual, you may join someone else’s project only if you have not submitted any entries for scoring during the contest’s current phase.
- No sharing. Sharing code between teams is prohibited unless the information shared is free and publicly available.
- Public discussion. You may not publicly describe the methodology you are using for the competition until after the submission deadline for a given phase. You may describe your methodology in the context of other datasets as long as you don’t also indicate this is the methodology you used in competition.
- Publication. Please use the recommended citation found on the dataset documentation page. Although the dataset is available to all Nightingale users, please do not submit contest-related work to other conferences or journals until the current contest has ended.
-
Scoring
- Predictions CSV. Teams submit entries for scoring in the form of a predictions file. Nightingale will score the entry according to the methodology described in the Scoring methodology section.
- Entry limit. Each team can submit 1 entry per day.
- Public leaderboard. During the competition period, each team’s current ranking will be visible on the competition public leaderboard. After the end date, the leaderboard will reveal each team’s score and model description.
- High score. The best score from all your team’s submissions will be used in the leaderboard, along with your description of that entry, if any. See Scoring for details.
- Tiebreaker. In the event of a tie, the first submission will outrank subsequent submissions.
- Submission requirements. Nightingale may make efforts to help you validate your predictions file and fix any issues before scoring. However, invalid submissions may result in no score and may count against your team’s entry limit.
-
Resources
- Use of non-public data or software. Use of free and publicly available external data is allowed, including pre-trained models. If you use any proprietary software or data that is not free and publicly available, such as a pre-trained model using private data, then you should declare this by including “[NON-PUBLIC]” in your Nightingale project description field. Rankings and scores for teams using non-public resources will be published on the leaderboard and encouraged to contribute to the conferences but will not be eligible for any prizes.
- Billing. Nightingale OS provides both free and non-free compute resources to contest participants. To support participants with the cost of non-free compute servers, Nightingale allocates a limited amount of free compute credits to the teams on sign up. Additional free compute credits will be allocated periodically based on availability of credits and each team’s activity. Teams are responsible for all additional costs incurred for non-free resources after they consume the free compute credits.
-
Prizes
- Review of entries and Deadlines. All top scoring entries are subject to Nightingale’s review for compliance with guidelines and quality of results. After the deadline, the organizers will add the reviewers to the leading projects so that their submissions can be reviewed for conformance with the rules for awarding prizes. Because the goal of this contest is to promote research and to stimulate interest in these kinds of applications, we could extend the deadline in order to get broader participation and high quality submissions. We will let everyone know in advance if we do change any key dates.
- Publishing and Licensing of work. To be eligible for prizes, the teams must publish their winning solution on their public Github code repository as open source under the Apache License v2 or an Apache-compatible open source license. The license must be added to your project files by the deadline to be eligible - see here for an example of how to do this. Third-party resources, if used, must be freely available to the public, and must not form a significant part of the solution as determined by the judges. This clause is intended to promote the repeatability and reproducibility of science. If a team is unable to comply with this clause, they may still compete and will be placed on the leaderboard, but they will not be eligible for prizes.
- Residency requirements. In accordance with NIH AIM-AHEAD policy, only US citizens and permanent residents are eligible to participate in this challenge and be eligible for prizes.
- Tax reporting and withholding. ALL TAXES IMPOSED ON PRIZES ARE THE SOLE RESPONSIBILITY OF THE WINNERS. Payments to potential winners are subject to the express requirement that they submit all documentation requested by competition organizers for compliance with tax reporting and withholding regulations. Prizes will be net of any taxes that the competition organizer is required by law to withhold. Foreign residents (as defined by US tax regulations) may be subject to mandatory 30% tax withholding. If a potential winner fails to provide any required documentation or comply with applicable laws, the Prize may be forfeited and the organizer may select an alternative potential winner. Any winners who are U.S. residents may receive an IRS Form 1099 in the amount of their prize. Any winners who are foreign residents may receive an IRS Form 1042-S in the amount of their prize and the tax amount withheld. <br id=”scoring” />
Scoring
Scoring methodology
Cancer stage takes on discrete values (0, I, II, III, IV), and clinically, some errors are more costly than others: in broad strokes, metastatic disease (stage IV) is managed very differently from locoregional disease, and carries a much higher mortality rate. We use the one-vs-rest weighted average AUC (area under the ROC-curve) to score the entries in this contest.
Nightingale has randomly sampled a set of patients to create a holdout set on which entries will be scored. You can see the images in the dataset directory in the holdout subdirectory, but of course, stage and mortality fields are excluded.
~/datasets/brca-psj-path/ndpi-holdout
To submit an entry, predict the stage for each biopsy and write results to a CSV file.
Predictions CSV file format
Although teams are being asked to use slides to train their algorithms, predictions for this contest are for biopsies. Each biopsy produces multiple slides. Teams will need to incorporate multiple slides into their algorithms or aggregate slide predictions at a biopsy level. Also be aware that biopsy_id, slide_id, and patient_ngsci_id all are hexadecimal strings of the same length.
-
Filename and location
-
The file needs to be located in your project directory. Specifically, a predictions file should be in
~/project
or any subdirectory. - The predictions file can have any name.
- For subsequent submissions, it doesn’t matter whether you use the same file or create a new one.
-
The file needs to be located in your project directory. Specifically, a predictions file should be in
-
CSV format
- The CSV file should have no header row.
- The Probability_Stage_x columns must each be floats between 0 and 1, inclusive.
- The total of the probabilities in each row must add up to 1.
- Predicted_stage must be an integer between 0 and 4, inclusive.
- Each line should have the following schema:
Column index | Contents | Data type |
---|---|---|
0 | Biopsy_ID | string |
1 | Probability_Stage_0 | float in range [0,1] |
2 | Probability_Stage_1 | float in range [0,1] |
3 | Probability_Stage_2 | float in range [0,1] |
4 | Probability_Stage_3 | float in range [0,1] |
5 | Probability_Stage_4 | float in range [0,1] |
6 | Predicted_Stage | integer in {0,1,2,3,4} |
Example CSV
47ba1eb2-0d3b-4752-80d3-6d318001751e,0.18312,0.67326,0.11121,0.03231,8.1357e-05,1
e4235769-c290-4bce-bf3a-9b98c7ef80b5,0.27895,0.25605,0.080242,0.38474,2.0360e-06,3
d9bd5e69-98ce-4736-a108-fd64234ffb05,0.0064058,0.16384,0.33646,0.32201,0.17126,2
...
note: no header is included
Submission description (recommended)
- You may optionally add a description for each submission. You don’t get to change the description later. We strongly recommend you use this field to note the version of the model that generated it, so that scores can be traced back to a particular model.
- You will see descriptions in your Submissions list inside your team’s Nightingale project.
- The public will see the description for your best submission after the contest end date.
Frequently Asked Questions
I’m new to Nightingale. How can I learn to use the platform quickly?
Please see the quick start tutorial here. The tutorial notebook is also available inside the platform - you can copy, run, and modify the notebook hands-on to learn to use the platform quickly.
How to request complimentary compute credits?
To request these compute credits, teams should submit a help request from the platform or email support@nightingalescience.org, mentioning their project id. Complimentary credits will expire at the of the contest. Please see the details on the amount of free compute credits here.
How to get additional paid compute credits?
If a team wants to pay for additional compute credits, they may generate an invoice for a specific dollar amount and have it mailed to the payee’s email address. This invoice email will contain an electronic link for online payment using a credit card. On successful payment, these credits will be added to the project. These paid compute credits will not expire at the end of the contest, and we are able to transfer unspent compute credits to a new project created by the same user.
How to submit an entry?
Submit your predictions file inside any instance in your Nightingale project:
>>> import ngsci
>>> ngsci.submit_contest_entry("path/to/your.csv", description="our model")
(<Result.SUCCESS: 1>, 'success')
After you submit a file, Nightingale will attempt to validate the schema of your CSV. If it fails validation, you will see one or more errors in the Submissions tab in your Nightingale OS project (not in your JupyterLab editor). Any validation errors detected automatically will not count against your submission limit.
After a predictions file passes validation, it will be submitted for scoring. The result will be posted in your project Submissions view, and if the result is a new high score for your team, the public leaderboard will also be updated.
Can I see a sample submission?
A sample CSV and notebook are located in ~/datasets/brca-psj-path/contest-phase-2/sample-submission
. The sample CSV is in the proper format and contains all the biopsy_id
‘s needed for a submission. The notebook describing the holdout tables and the process of submitting an entry.
How should I submit my model?
Teams that are in contention for winning prizes will be expected to share their projects with Nightingale staff within 3 days after the submission deadline. More precise instructions will be given to the top teams a week before the deadline.
Expected to include:
- The notebooks or scripts used to train the model
- The weights or serialized model used for winning submission, which can be used to reproduce submission results
- A Open-source license
Here are some tutorials for saving weights and models in PyTorch and Tensorflow.
How to enter the contest and form a team?
After you have registered for Nightingale and a Nightingale admin has admitted you, then you can enter the currently active contest. For example: Predicting High Risk Breast Cancer Phase 2 - 2023
.
When you enter the contest, a new, empty project is created for you and associated with this contest. This project is your team workspace.
- Your project (team) name and description will be publicly visible on the leaderboard.
- Add teammates by making them member of your project. We strongly suggest that each team include some members from communities underrepresented in data science and artificial intelligence and machine learning. For examples, see here.
- Only registered users are eligible to be added to your project/team.
- You can only be a member of one team per contest.
How to transfer data from a Phase 1 contest project into a Phase 2 project?
If you worked on Phase 1, you probably want to use some of the project files that you created in your new Phase 2 project. There isn’t a direct way to copy from one project to another because each Nightingale OS project is isolated. You can migrate project data, however, using your home directory.
- Start an instance in the Phase 1 project.
-
Copy data that you want to transfer from the (shared)
${HOME}/project
directory to your (private)${HOME}
directory. - Stop your instance in the Phase 1 project.
- Start an instance in the Phase 2 project.
-
Move or copy the data that you want to transfer from
${HOME}
to the (new)${HOME}/project
directory.
Host organizations
This is a challenge jointly hosted by NIH AIM-AHEAD Data Science Training Core and Nightingale Open Science.
Nightingale Open Science is a platform connecting researchers with deidentified, cutting edge medical datasets. The Nightingale OS team works closely with health systems around the world to create and curate datasets of medical images linked to ground-truth labels, and make them freely available to academic researchers. Nightingale OS launched at the 2021 NeurIPS conference with five anchor datasets spanning different disease areas.
The National Institutes of Health’s Artificial Intelligence/Machine Learning Consortium to Advance Health Equity and Researcher Diversity (AIM-AHEAD) program has established mutually beneficial, coordinated, and trusted partnerships to enhance the participation and representation of researchers and communities currently underrepresented in the development of AI/ML models and to improve the capabilities of this emerging technology, beginning with electronic health records (EHR) and extending to other diverse data to address health disparities and inequities.
Providence St. Joseph Health is a not-for-profit health care system operating in seven states and serves as the parent organization for 100,000 caregivers. The combined system includes 51 hospitals, 829 clinics, and other health, education and social services across Washington, Oregon, California, Alaska, Montana, New Mexico, and Texas. The data set for this challenge was contributed by Providence St. Joseph Health.
Acknowledgements
We thank our generous funders: The NIH funded AIM-HEAD Data Science Training Core, Schmidt Futures, The Gordon and Betty Moore Foundation, and Ken Griffin. We would also like to acknowledge the team that created and conceived of this dataset, and worked with us to make this challenge possible: Carlo Bifulco, MD, Director of Molecular Pathology and Pathology Informatics; Brian Piening, PhD, Technical Director of Clinical Genomics; Tucker Bower, Bioinformatics Scientist. Many thanks also to the leadership of Ari Robicsek, Chief Medical Analytics Officer at Providence, Bill Wright, VP of Health Innovation at Providence, and Raina Tamakawna, Enterprise and GME Research Program Manager at Providence.
We express our thanks to Hamamatsu as well – developers of the NanoZoomer 360 platform, Hamamatsu supported this work with a grant from their Product Marketing Division and partnered with Providence to ensure a seamless start to the dataset creation process.
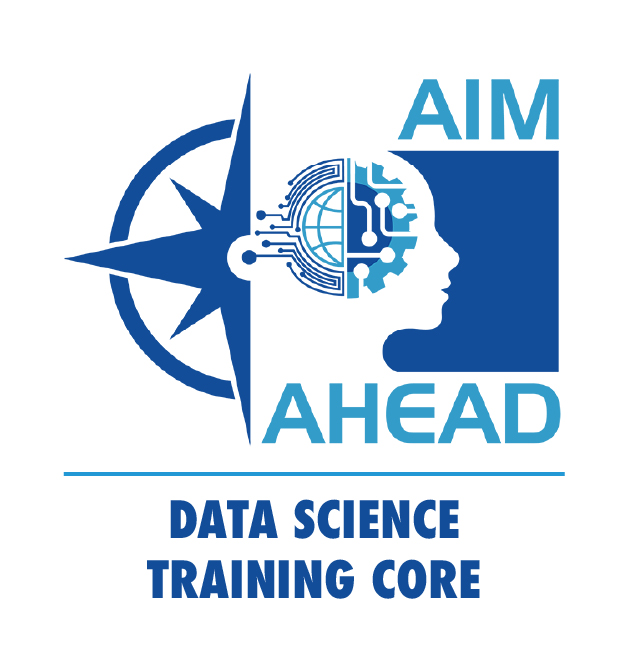
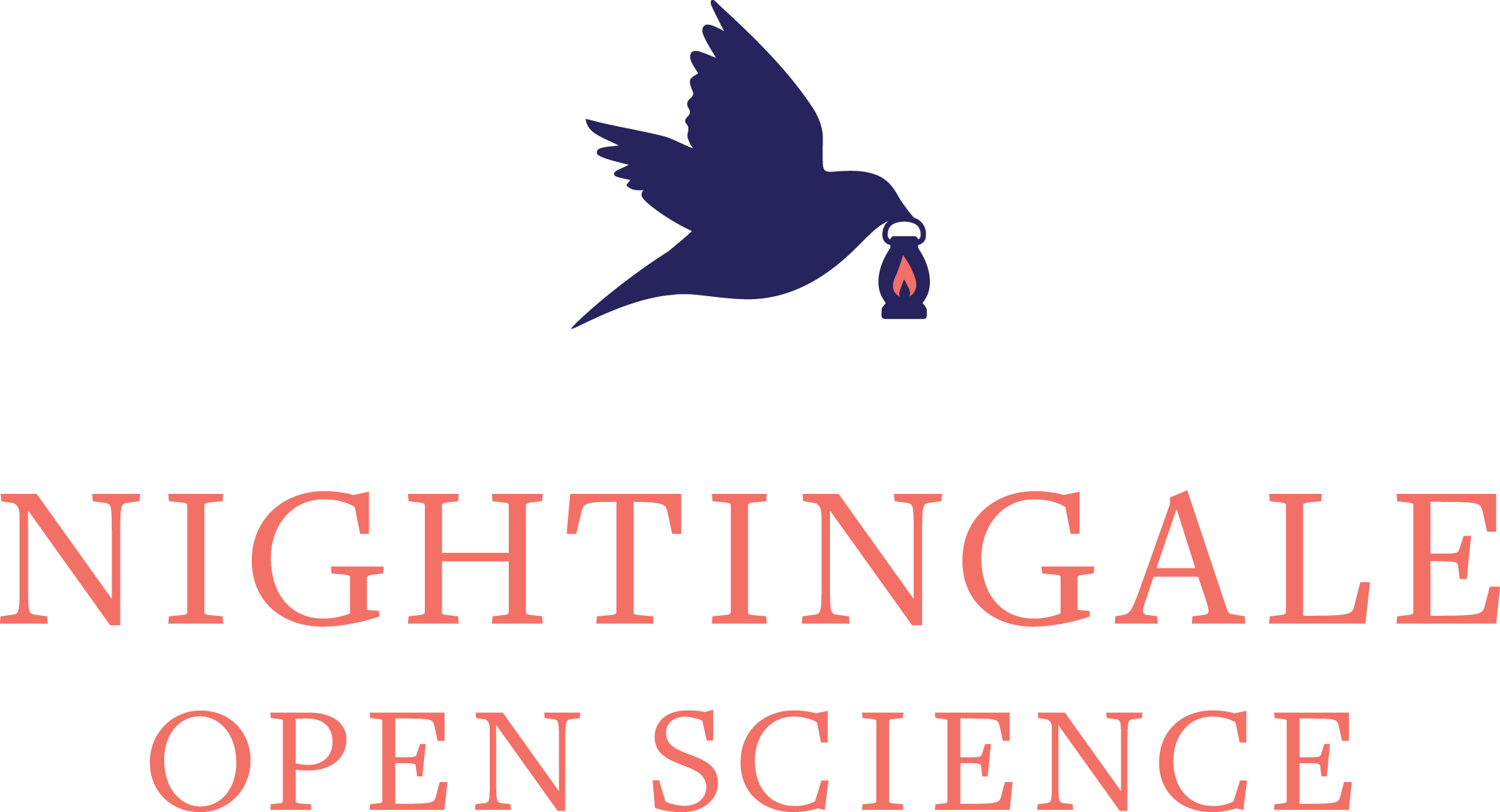